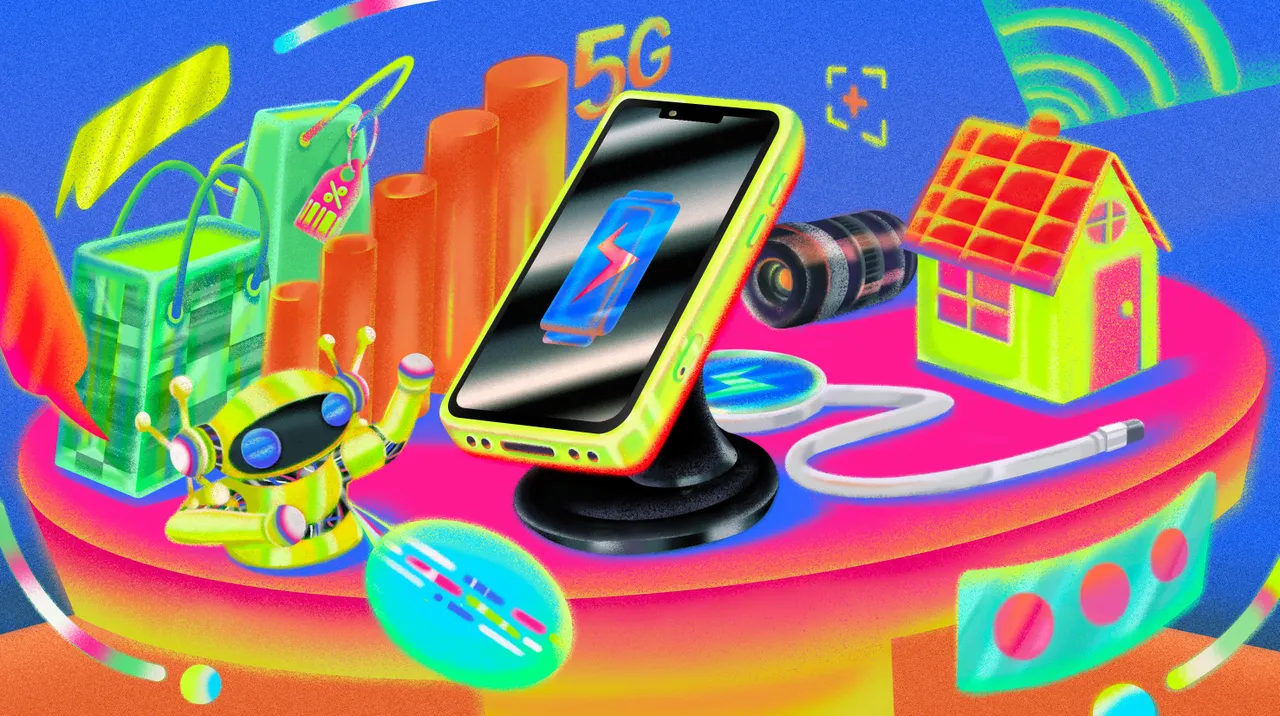
The Role of Artificial Intelligence in Shaping the Future of Mobile Technology
When it replaces rote work and processes to cut costs for business entities, and frees up workers to use their uniquely human capacities.
Proponents look forward to a world of AI that complements rather than substitutes work, enhancing productivity and sharing the fruits of greater prosperity; opponents fear that AI could aggravate surveillance even as it consolidates the growing fortunes of the global elite.
Artificial Intelligence
A broad and varied set of technologies that performs tasks that have traditionally been undertaken by at least some humans – encompassing everything from data entry to factory robots to chatbots and self-driving cars.
Modern AI first emerged with Marvin Minsky and John McCarthy’s landmark ‘Logic Theorist’ computer program in 1956 – a year many now consider a birth date for the modern field. Soon thereafter, AI researchers divided the field into four subtypes ranging from systems focused on single tasks, many of which we now routinely use, to sentient machines that don’t yet exist.
It is increasingly seamlessly embedded in manufacturing costs and worker safety reduction, facilitating patient diagnosis and treatment, speeding drug discovery, identifying patterns in mountains of data, and even providing chatbots and banking customer service systems, all of which are increasingly tailoring transactions to customers while reducing compliance costs and improving the management of risk.
Machine Learning
The key to AI is machine learning, in which an algorithm learns from data to get its job done, rather than always doing the same thing in the same way. It’s the program called up by the data scientist ‘over and over again’ in the preceding quote, that adjusts according to its experiences that result in better performance in the future.
To identify objects, or track a fast-moving vehicle, an AI system might learn by processing a sequential stream of data (for instance, from visual images or a lidar sensor), while trying to understand language might involve encoding the statistical distribution of characters into a model. To achieve these tasks, data are interpreted and translated into an algorithm that guides the behaviour of the machine, helping it gradually become more capable over time.
Groundbreaking work from researchers such as Alan Turing first suggested that machines might be truly intelligent in the 1950s and ’60s, with early accomplishments in AI including GPL (general problem solver) algorithm from Newell ́s Simon, LISP (list processing) programming language developed by John McCarthy, and ELIZA – an early natural language processing simulation that tried to imitate conversation by Joseph Weizenbaum.
It underlies computer search engines, as well as voice and handwriting recognition software; it’s at the core of medical diagnostics and robotic surgery, financial industry fraud detection systems, and the market-pattern analysis that helps manage buying and selling of stocks; and it’s also what allows retailers to automate aspects of their marketing strategies and customer support.
Natural Language Processing
Natural Language Processing, on the other hand, processes speech and text the way people do: using rules and algorithms to interpret, bit by bit, what a speaker or a piece of writing actually means, flexibly, fluidly and full of nuance. This is particularly an endeavour in human differences: NLP makes millions of computations, taking in real-world input from sensors such as cameras or microphones and coding, interpreting and analysing information in order to make sense of it.
NLP will have the ability to scan large swathes of data to uncover nuanced patterns that could take a human formatter weeks of manual analysis to discover. NLP can also automate repetitive forms of cognitive labour to help workers direct their energy towards more meaningful work.
EEG is also used in classrooms to discern the emotions of individual participants and adapt learning accordingly. Daily, banks and financial institutions can employ EEG to detect fraud, audit accounts and scrutinise loan applications.
We’ve already acknowledged the role of AI in customer service and identifying viable candidates for recruitment. What impact has AI-related automation had on employment as a whole? Some critics worry that jobs will be replaced or eliminated by artificial intelligence, even as others observe that such technologies will increase productivity in hopes of creating new jobs.
Deep Learning
Deep learning (a specific type of ‘machine learning’) enables neural networks to process optical images and video, and thereby recognise things in the world and parse natural-language objectives. Deep learning provides the foundation for many smartphone-based AI assistants, search engines, self-driving cars and recognition of human speech or handwriting in many other apps.
If the 1950s and ’60s brought ambitious forecasts from AI’s pioneers, they also brought torrents of government and industry investment, now widely held up as an exemplar of the dangers of short-sighted bureaucracy. The early, ambitious efforts – such as the Newell & Simon General Problem Solver – only scraped the surface, but did pave the way for things to come: McCarthy had invented an AI-tailored programming language called Lisp which remains in wide use to this day.
Businesses in every sector are resorting to AI for decisions or tasks that would otherwise be humanly impractical or infeasible including AI-powered chatbots that offer customer service, robotics in manufacturing with systems that monitor workflows in factories and other similar industries, and in financial services where it is widely used for instance to recognise the probability of fraud, for credit scores and to manage trading in stock markets based on historical trends, or to flag up fraudulent transactions.